Artificial Intelligence: India
Line 9: | Line 9: | ||
+ | |||
+ | =History= | ||
+ | ==1986- 1993: Research and development== | ||
+ | [https://epaper.indiatimes.com/article-share?article=16_03_2024_203_018_cap_TOI March 16, 2024: ''The Times of India''] | ||
+ | |||
+ | |||
+ | The recent breakthroughs by LLMs have captured everyone’s imagination. These LLMs are the pinnacle of the connectionist AI paradigm that trains neural networks to recognise patterns in data to solve problems. The access to massive amounts of digital annotated data thanks to the internet and immense computing power on the cloud have made the connectionist paradigm popular now. The symbolic AI paradigm that used prior knowledge, logical inference, and rules to solve problems was popular towards the end of the 20th century. | ||
+ | |||
+ |
Projects in 3 domains | The government of India, with assistance from the United Nations Development Programme initiated the Indian Fifth Generation Computer Systems / Knowledge Based Computer Systems (FGCS/KBCS) programme in 1986. The name was inspired by the ambitious Japanese national Fifth Generation Computer Systems R&D programme in that era whose objective was to create computing systems to provide a platform for future developments in artificial intelligence by the early 1990s and the symbolic AI paradigm. The FGCS/KBCS programme had a bouquet of interesting R&D projects in three domains – parallel processing platforms, language processing technologies, and applications of knowledge-based computer systems. | ||
+ | |||
+ |
IISc, C-DAC work | R&D in parallel processing systems anchored by IISc and C-DAC focused on building parallel computers with different architectures on which the AI applications developed by other FGCS/KBCS R&D teams would run. The IISc team worked on multidimension multi-link systems, tree architectures, and coarse-grain static dataflow multiprocessors. They also developed systems software for these multiprocessor systems. C-DAC’s focus was on developing a parallel processing platform, and a graphics and intelligencebased systems technology for Indian language processing. | ||
+ | |||
+ |
Language processing tech | TIFR and National Centre of Software Technology (NCST) anchored the R&D in language processing technologies. The TIFR team explored subword unit-based speech recognition using clustering and statistical modelling techniques to obtain an inventory of subword units. They established a correspondence between subword units and linguistic units. They developed hidden Markow models-based word recognisers for recognising digits and words for application in a railwaysrelated enquiry task. Acoustic phonetic features for speech recognition were the focus of another R&D project. The overall aim was to use the hidden Markow models and acoustic phonetic features to build a speech recognition system for handling a railways-related enquiry task in Hindi. The TIFR team also experimented with the connection- ist paradigm including back-propagation techniques and developed a neural network for speech recognition. They developed a single-speaker digit recogniser. The NCST team worked on machine translation from English to Hindi. | ||
+ | |||
+ |
Knowledge applications | The applications of knowledge-based computer systems were the focus of R&D at IIT Madras and the government’s department of electronics (DoE). IIT Madras developed an expert information system in healthcare that could diagnose twenty-three ailments using a fuzzy decision-oriented methodology. The other features of the system were a family guide for common ailments, a training system for medical staff, and a voice-input enquiry system for recommending medi- cines. This system was for use in a non-urban context where there was a paucity of doctors. | ||
+ | |||
+ |
Projects in manufacturing | Another R&D project at IIT Madras developed expert systems in manufacturing contexts. These included a process planning system to make cold forged fasteners, and a selection system for drill and cutting tools. An interesting R&D project at IIT Madras was an expert system for the range safety officer during a rocket launch. DoE built an expert system to assess the income tax of an individual taxpayer. This system modelled all the existing rules and the expert heuristics used at that time for assessing items like conveyance, accommodation, etc. Another project of DoE represented the prevalent import and export policy and procedures as a knowledge tree. | ||
+ | |||
+ |
Devanagari script for PCs | Some of the R&D projects in the FGCS/ KBCS programme translated their research into applications. C-DAC’s work on graphics and intelligencebased systems technology for language processing resulted in making Devanagari script available for PCs. DoE’s projects on an expert system for income tax assessment and import policies had pilot implementations. The NCST implemented an intelligent information archival and retrieval system for a leading wire service agency.
| ||
+ | |||
+ | ''' Rich legacy ''' | ||
+ | |||
+ |
We have a rich legacy in socially impactful AI R&D and have traversed a long way since the 1980s. Indian AI talent and R&D are world-class. We have an impressive bouquet of state-of-the-art and socially relevant public-funded AI R&D programmes. We must translate robust and safe outcomes from our AI R&D into products and solutions that feed into the Indian digital public infrastructure. The Bhashini initiative is one example. | ||
=Nature Index= | =Nature Index= | ||
Line 41: | Line 64: | ||
ARTIFICIAL INTELLIGENCE: INDIA]] | ARTIFICIAL INTELLIGENCE: INDIA]] | ||
[[Category:S&T|A | [[Category:S&T|A | ||
+ | ARTIFICIAL INTELLIGENCE: INDIA]] | ||
+ | |||
+ | [[Category:India|A ARTIFICIAL INTELLIGENCE: INDIA | ||
+ | ARTIFICIAL INTELLIGENCE: INDIA]] | ||
+ | [[Category:Pages with broken file links|ARTIFICIAL INTELLIGENCE: INDIA]] | ||
+ | [[Category:S&T|A ARTIFICIAL INTELLIGENCE: INDIA | ||
ARTIFICIAL INTELLIGENCE: INDIA]] | ARTIFICIAL INTELLIGENCE: INDIA]] |
Latest revision as of 20:05, 24 March 2024
This is a collection of articles archived for the excellence of their content. |
Contents |
[edit] History
[edit] 1986- 1993: Research and development
March 16, 2024: The Times of India
The recent breakthroughs by LLMs have captured everyone’s imagination. These LLMs are the pinnacle of the connectionist AI paradigm that trains neural networks to recognise patterns in data to solve problems. The access to massive amounts of digital annotated data thanks to the internet and immense computing power on the cloud have made the connectionist paradigm popular now. The symbolic AI paradigm that used prior knowledge, logical inference, and rules to solve problems was popular towards the end of the 20th century.
Projects in 3 domains | The government of India, with assistance from the United Nations Development Programme initiated the Indian Fifth Generation Computer Systems / Knowledge Based Computer Systems (FGCS/KBCS) programme in 1986. The name was inspired by the ambitious Japanese national Fifth Generation Computer Systems R&D programme in that era whose objective was to create computing systems to provide a platform for future developments in artificial intelligence by the early 1990s and the symbolic AI paradigm. The FGCS/KBCS programme had a bouquet of interesting R&D projects in three domains – parallel processing platforms, language processing technologies, and applications of knowledge-based computer systems.
IISc, C-DAC work | R&D in parallel processing systems anchored by IISc and C-DAC focused on building parallel computers with different architectures on which the AI applications developed by other FGCS/KBCS R&D teams would run. The IISc team worked on multidimension multi-link systems, tree architectures, and coarse-grain static dataflow multiprocessors. They also developed systems software for these multiprocessor systems. C-DAC’s focus was on developing a parallel processing platform, and a graphics and intelligencebased systems technology for Indian language processing.
Language processing tech | TIFR and National Centre of Software Technology (NCST) anchored the R&D in language processing technologies. The TIFR team explored subword unit-based speech recognition using clustering and statistical modelling techniques to obtain an inventory of subword units. They established a correspondence between subword units and linguistic units. They developed hidden Markow models-based word recognisers for recognising digits and words for application in a railwaysrelated enquiry task. Acoustic phonetic features for speech recognition were the focus of another R&D project. The overall aim was to use the hidden Markow models and acoustic phonetic features to build a speech recognition system for handling a railways-related enquiry task in Hindi. The TIFR team also experimented with the connection- ist paradigm including back-propagation techniques and developed a neural network for speech recognition. They developed a single-speaker digit recogniser. The NCST team worked on machine translation from English to Hindi.
Knowledge applications | The applications of knowledge-based computer systems were the focus of R&D at IIT Madras and the government’s department of electronics (DoE). IIT Madras developed an expert information system in healthcare that could diagnose twenty-three ailments using a fuzzy decision-oriented methodology. The other features of the system were a family guide for common ailments, a training system for medical staff, and a voice-input enquiry system for recommending medi- cines. This system was for use in a non-urban context where there was a paucity of doctors.
Projects in manufacturing | Another R&D project at IIT Madras developed expert systems in manufacturing contexts. These included a process planning system to make cold forged fasteners, and a selection system for drill and cutting tools. An interesting R&D project at IIT Madras was an expert system for the range safety officer during a rocket launch. DoE built an expert system to assess the income tax of an individual taxpayer. This system modelled all the existing rules and the expert heuristics used at that time for assessing items like conveyance, accommodation, etc. Another project of DoE represented the prevalent import and export policy and procedures as a knowledge tree.
Devanagari script for PCs | Some of the R&D projects in the FGCS/ KBCS programme translated their research into applications. C-DAC’s work on graphics and intelligencebased systems technology for language processing resulted in making Devanagari script available for PCs. DoE’s projects on an expert system for income tax assessment and import policies had pilot implementations. The NCST implemented an intelligent information archival and retrieval system for a leading wire service agency.
Rich legacy
We have a rich legacy in socially impactful AI R&D and have traversed a long way since the 1980s. Indian AI talent and R&D are world-class. We have an impressive bouquet of state-of-the-art and socially relevant public-funded AI R&D programmes. We must translate robust and safe outcomes from our AI R&D into products and solutions that feed into the Indian digital public infrastructure. The Bhashini initiative is one example.
[edit] Nature Index
[edit] 2020
Chandrima Banerjee, December 26, 2020: The Times of India
Elephants don’t play chess. They don’t need to. They need to know how to forage for food, protect themselves from predators, mate and migrate. In 1990, this argument changed how the world thought of Artificial Intelligence. MIT professor Rodney Brooks said AI must interact with the physical environment and not just rely on complex (and slow) computation of symbols, the way human brains do. So, they don’t all need to play chess.
Three decades since then, AI seems to be in its summer (periods of slow funding have been called ‘AI winter’ by researchers). Between 2000 and 2019, global output for AI research grew by more than 600%, the latest ‘Nature Index 2020 Artificial Intelligence’, released last week, said. China, with a 120% jump in output, leads AI research this year. That has been complemented by technology we can use. The Nature Index placed the US at the top for AI innovation this year.
India has been the third most productive country in AI research, with over 23,000 papers. On the overall AI Index, it is at the 20th position in alist dominated by European countries. Of the top 100 research organisations Nature identified, only one Indian organisation made the cut — Anna University in Chennai.
In fact, an AI Readiness Index by UK-based consultancy Oxford Insights had in September given India a score of 100 on vision. Yet, the country was 40th on a list of 172 countries. “India, Russia and China all score near the bottom of the (Responsible Use) Sub-Index,” it said. So, it gave India a score of 31 on privacy and just 23 on transparency.
[edit] Startups
[edit] 2022
Shilpa Phadnis, June 23, 2023: The Times of India
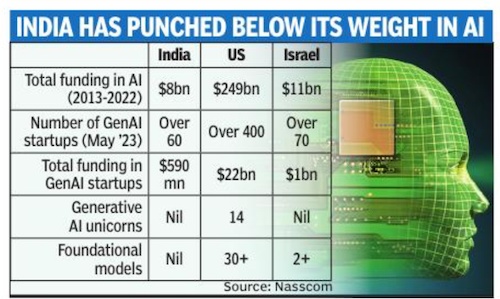
From: Shilpa Phadnis, June 23, 2023: The Times of India
BENGALURU: India has over 60 generative AI startups, says a new report by industry body Nasscom. Generative AI startups in the country, it said, have already raised $590 million in funding, and most of it ($475 million) was from 2021 alone.
The Bengaluru region has 45% of the generative AI startups. The city's deeptech startup ecosystem, high-end innovation-driven institutions, extensive industry presence and an emerging class of domestic angel investors are seen to be a big draw. The Mumbai-Pune region accounts for the second-largest pool, at 21%. This region boasts of some of the most well-established institutional investors & VCs, and a diverse talent pool.
The report said 74% of startups are generative AI native and 26% are those that have pivoted. About 37% of the non-commercialised solutions are expected to find markets within a year. Many Indian generative AI startups prefer a tech stack comprising public cloud and cloud-based databases, pre-trained models, and custom-built visualisation tools.
"Being a nascent technology, big-ticket investments, particularly in native Indian foundational models and enterprise-grade applications services, are yet to happen. In addition to limited investments, generative AI startups in India also face the challenge of limited high-quality and ready-to-use training datasets and lack of high-performance compute capacity at scale. Lack of clarity on data privacy, security, ethical guidelines, and globally consistent generative AI usage standards can further slow down growth," Nasscom executives Sangeeta Gupta, SVP & chief strategy officer, and Achyuta Ghosh, research head, say in the report. They said companies will find it difficult to upskill a large workforce rapidly in 6-12 months.
Nasscom's data shows that $8 billion was pumped into AI from 2013 to 2022 ($3.2 billion in 2022 alone) across 1,900 AI startups in India.